3.3
Hyperparameters, Cultural Luthiery, and Sacred Tuning Systems
︎ Refer to Appendix A8 for ancillary musical, historical, and technical details
Even in their most transparent states these algorithms are opaque mechanisms that elude even the mathematicians that have constructed them. We shovel exorbitant amounts of data into their inputs to arrive at some result on the output. But what goes on inside these black boxes? The answer is that we really do not exactly know how these algorithms arrive at the output. We know the principles for how they process data but there isn't a rationalizing component as to why or how it provides its results. Most companies treat these types of heuristics as probability oracles. By espousing market-based ideologies they can afford their calculations to be off by a certain percentage because by playing the long game of probability they will be correct most of the time. But what about those times when its not accurate? When it means that you sent an advertisement to someone who isn't interested — that's just a regression problem that will eventually be corrected for with more data, they say. But what if it is calculating recidivist risk75, which neighborhoods to patrol76, or who should be deported77? We cannot afford to treat these heuristics as objective and they cannot be entrusted to opaque interests. In their current state these algorithms give us results but are incapable of explaining their reasoning.78 This is why we need justifiable AI, or AI that ‘shows its work’ to justify how it arrived at its conclusion.
Former Facebook engineer Carlo Bueno has said that “when you quiz a technical person about how to create justifiable AI, their usual gut reaction is to publish the data and stats. How can you argue with something that seems to work? You can’t prove how something will behave in the big bad old world until you put it out in there. And purely statistical arguments are not compelling to most of the public, nor are they usually sufficient under the law. Means and motive matter as much as ends. AIs don’t operate in isolation. Somebody designs them, somebody gathers the data to train them, somebody decides how to use the answers they give. Those human scale decisions are — or should be — documented and understandable, especially for AIs operating in larger domains for higher stakes. It’s natural to want to ask a programmer how you can trust an AI. More revealing is to ask why they do.”79
What does responsibility even look like when it comes to these types of technologies? Firstly, making sure that there isn't a single (invisible) hand on the “tuning pegs” for these algorithms. Public oversight and decentralized infrastructure projects are mission critical for steering these narratives away from high risk scenarios. The sooner that we can move towards a public literacy on these topics, the sooner we can move toward options for common interest.
Co-operative parametric design is my preferred term for democracy 2.0; voting systems for recalibrating the different facets of society, labor forces, and cultural apparatus. Democracy is slow because it requires consensus. Polling people once every few years with the aim of having a representative interpret your interests has been left outmoded by the high frequency algorithmic learning processes of technocratic market design. Co-operative parametric design is an idea that operates as a scientific management layer for implementing bottom-up decision making to modify parameters at the rate that our world moves at. This concept should also be paired tightly with distributed ownership over the algorithms and potentially quadratic voting.80 As I mentioned in the first chapter, platforms address an issue of scale that, in their current state, tend to be authoritarian. However, this doesn’t have to be inherent to how platforms operate. It may be hard to envision a platform that isn’t monolithic because we don’t currently have another model but it is technically feasible with distributed systems design and decentralized infrastructure initiatives.
In recent years there have been many improvements in asynchronous training specifically with back propagation that leverage opt-in distributed computing infrastructure to counteract the centralization in deep learning training.81 The Berkeley Open Infrastructure for Network Computing, informally known as BOINC82,
is currently using opt-in resources to run LHC@Home CERN83 (European Organization for Nuclear Research) and Berkeley’s SETI@Home84 initiative for analyzing radio telescope data from space. However, the issues for a parametric co-operative approach like this aren’t only technical, they are primarily political.
Secondly, there needs to be substantial critical research on how these algorithms actually arrive at their results. Testing in a marketplace isn't sufficient data science for applications that exist outside of a market. Decreasing the marginal statistical outliers are not sufficient. Coming close isn't good enough. Not with AI. We need AI that can show its work. We need a right to explanation for algorithmic decisions.85 We need transparency86 and justifiable AI. From a policy standpoint this means we need a formalized consensual process of data acquisition. What does this mean from a technical standpoint? A few examples of the logistical “tuning pegs” in these complex systems that could refactor the heuristic biases lack of robust probability would include an examination of methodological underdetermination87 and metaphysical overdetermination88 within these systems.
Thirdly, we need to shift from the data-as-capital model to a data-as-labor model. What does this mean? Platforms are currently designed to siphon their users’ data to train their algorithms. For example, Uber is using their drivers’ GPS locations to train AI for their self-driving vehicles. These drivers are effectively training their replacements resulting in unemployment and increasing surplus populations. Google, Facebook, and others are free services because they are able to scrape information about its users and sell it to their clients. These are two examples of data-as-capital. The alternative, data-as-labor, might look like shifting to a cooperative platform where worker-owners not only steer the interests and applications of the algorithms, but are also afforded a dividend on their contribution to the data sets.
This is critical for a number of reasons. First of all, laborers become partial owners of the company by providing labor as a service to the company that is tracking them, or they have the option to easily opt out. Secondly, by the time that full automation comes about and these companies no longer need human labor to train their models the former labor markets have partial stake in the company and can expect returns on the work contributed to building an automated system. These initiatives for dividends need to be careful not to short change themselves and accept meager scraps from the technocracy, but couple these programs with distributed ownership as well. The former without the latter is moderate economism that will only deepen the rampant neoliberalism in suffused in Silicon Valley. Evgeny Morozov aptly describes the risk of such tactics stating that “treating data as a commodity would also make non-market solutions infeasible and costly. Imagine a resource starved city hall aspiring to build an algorithmic system for coordinating mobility services. On discovering that it now needs to pay for the data of the residents, it might never proceed with the plan. Deep pocketed firms like Uber do not face such hurdles.”89
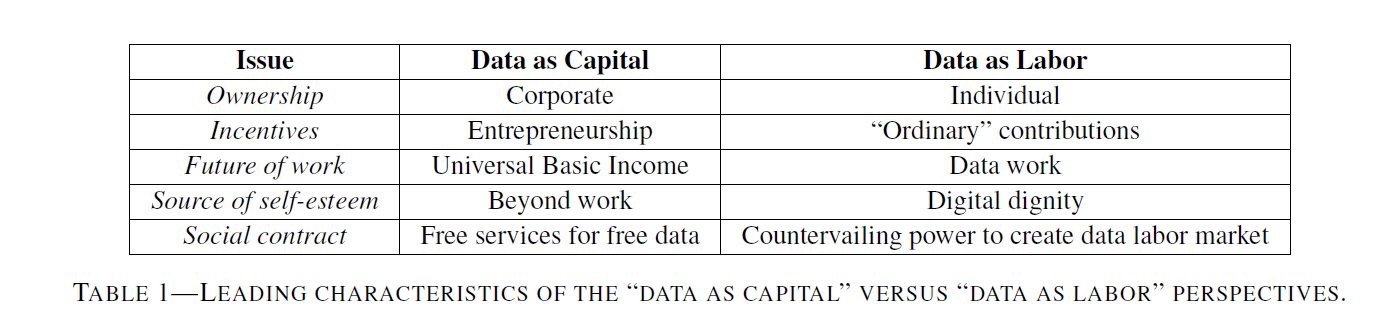
["Should We Treat Data as Labor? Moving Beyond 'Free'" American Economic Association Papers & Proceedings, 2017. Image Credit: Imanol Arrieta Ibarra, Leonard Goff, Diego Jiménez Hernández, Jaron Lanier, E. Glen Weyl] 90
In conjunction with corrective legislative and political updates, protocol layer technical rebuttals to the vectoralist systems of extraction will be required. For those who are contributing music, art, videos, design, photos, and other creative work (now cherishingly classified as ‘content’) to the platform aggregators there needs to be some sort of value exchange between the service provider and the content creator outside of the meager royalty model. The long game of these companies is to be able to generate content with deep learning instead of paying artists. Without equity in the platform and dividend returns on contributions this isn’t an optimistic future for the creative industries. For those that believe that creativity is the last bastion of humanity, consider that Disney is already generating scripts with AI91 and Warner Brothers just signed a major record deal with an algorithm.92
Admittedly, both platform co-ops and data-as-labor need a considerable amount of tuning and hardening to be able to adequately address the issues at hand, but could serve as a template for migrating to consonant relations. If these type of collectivist coordination schemas are to succeed, narrowly modeling threat and risk sets for the future solely around pre-existing algorithms is setting the sights too low given the amount of emerging venture capital backed companies that are positioned to vertically dominate entire markets. The preeminent quandaries that these co-operative initiatives need to address are the externalized costs of platform development and the geopolitical predicament of ‘membership’ or citizenry.
75 "Recidivism Risk Assessments Won't Fix the Criminal Justice System" https://www.eff.org/deeplinks/2018/12/recidivism-risk-assessments-wont-fix-criminal-justice-system
76 "Artificial Intelligence Is Now Used to Predict Crime." https://www.smithsonianmag.com/innovation/artificial-intelligence-is-now-used-predict-crime-is-it-biased-180968337
77 "Amazon is the invisible backbone behind ICE's immigration crackdown. - MIT" https://www.technologyreview.com/s/612335/amazon-is-the-invisible-backbone-behind-ices-immigration-crackdown
78 "Explainable Artificial Intelligence." https://www.darpa.mil/program/explainable-artificial-intelligence
79 "Justifiable AI - Ribbonfarm." https://www.ribbonfarm.com/2018/03/13/justifiable-ai/
80 "Quadratic Voting - School of Social Science." https://www.sss.ias.edu/files/pdfs/Rodrik/workshop%2014-15/Weyl-Quadratic_Voting.pdf
81 "Donate Your Computer's Spare Time to Science - The New York Times." https://www.nytimes.com/2016/10/20/technology/personaltech/donate-your-computers-spare-time-to-science.htm
82 "BOINC@berkeley." https://boinc.berkeley.edu
83 "LHC@home - CERN." http://lhcathome.web.cern.ch
84 "SETI@home - UC Berkeley." https://setiathome.berkeley.edu
85 "European Union Regulations on Algorithmic Decision-Making" https://www.aaai.org/ojs/index.php/aimagazine/article/view/2741
86 "About - AlgoTransparency." https://algotransparency.org/methodology.html
87 "Underdetermination of Scientific Theory (Stanford)" https://plato.stanford.edu/entries/scientific-underdetermination/
88 "The Metaphysics of Causation (Stanford)" https://plato.stanford.edu/entries/causation-metaphysics/
89 "The left needs to get radical on big tech – moderate solutions won't cut it." https://www.theguardian.com/commentisfree/2019/feb/27/left-radical-big-tech-moderate-solutions
90 "Should We Treat Data as Labor? Moving Beyond 'Free'" https://papers.ssrn.com/abstract=3093683
91 "Generating Animations from Screenplays." https://arxiv.org/abs/1904.05440
92 "Endel" http://endel.io/